What is generative AI?
Generative AI (GenAI) is a subset of artificial intelligence (AI) that can create (generate) text, images, audio, or video when prompted. It uses specific machine learning (ML) models, also known as deep learning, to analyze large data sets, recognize patterns, and generate content.
An essential guide to generative AI
Imagine a semiconductor manufacturer using generative AI to analyze wafers, optimizing yield before production even starts. Picture an oil & gas engineer applying AI-generated geospatial data to predict drilling success. Or envision researchers in life sciences using generative AI to analyze clinical trial scenarios, accelerating drug development and improving patient outcomes.
These are not hypothetical scenarios. They are real-world applications of generative AI that demonstrate its transformative power across industries. For data-driven professionals—analysts, domain experts, and business intelligence leaders—understanding generative AI is vital. Here’s a look at its core concepts, differences from traditional artificial intelligence, and implications for your workflows.
What is generative AI?
Generative AI refers to systems capable of creating new data that mimics existing patterns. Unlike traditional AI models that classify or predict, generative AI produces content, such as images, text, audio, code, or even data tables. Examples include OpenAI's GPT for text generation, DALL·E for image creation, and algorithms that synthesize realistic data for simulations or analytics.
Here are some examples of industry use cases:
- High-tech manufacturing: Simulating defect patterns or optimizing production line designs.
- Oil & gas: Creating synthetic geospatial datasets for reservoir analysis.
- Life sciences: Modeling patient populations to predict outcomes in clinical trials.
Generative AI versus traditional AI
Traditional AI is trained to recognize patterns or make decisions based on inputs. For example, an AI-powered credit card fraud detection system may analyze transaction patterns to classify whether a purchase is legitimate or fraudulent.
Generative AI, on the other hand, goes beyond analysis, actively creating new outputs. While traditional AI answers "what might happen," GenAI asks, "what can we create or analyze?"
The Spotfire Copilot AI tool takes generative AI one step further for Spotfire users. The GenAI extension can even generate charts or data functions when prompted. This goes beyond just text and image generation to code and analysis generation. The Copilot can provide instructions on how to create a data function in Spotfire, or even create a specific reusable data function once it understands what the user is trying to achieve in an analysis.
Traditional AI |
Generative AI |
|
Purpose |
Predict or classify outcomes |
Generate new, realistic data |
Output |
Labels, trends, predictions |
Text, images, synthetic datasets, visualizations, data functions |
Examples |
Forecasting production delays |
Discovery of hidden patterns of production issues |
Why generative AI matters for businesses
Enhanced decision-making: With generative AI, professionals can analyze scenarios, create synthetic data to test models, or develop hyper-personalized customer experiences.
Improved efficiency: Automation capabilities reduce manual effort and time. For instance, the Spotfire Copilot AI tool can assist Spotfire users by crafting customized visualizations from multiple data tables or sources—or accelerating data preparation tasks when prompted.
Innovation at scale: Unlock creative and data-driven potential, from designing new products to transforming customer interactions with AI-generated content.
Best practices for integrating generative AI
- Start small, think big: Begin with manageable pilot projects that address specific business needs, such as generating synthetic datasets to enhance model training. Use these successes to build internal confidence and identify broader applications.
- Leverage the right tools: Invest in platforms and tools that integrate seamlessly with your existing workflows.
- Focus on data quality: Generative AI's output is only as good as the data it learns from. Regularly assess and clean your datasets to ensure they are accurate, diverse, and representative of your problem space.
- Upskill your team: Equip your workforce with the necessary knowledge and skills to maximize the benefits of generative AI. Offer training on AI fundamentals, tools like Python and R, and domain-specific applications to empower data professionals.
- Prioritize ethical AI usage: Implement safeguards to ensure responsible AI adoption. This includes bias mitigation in data inputs, transparency in how AI models operate, and regular audits to maintain trust and compliance with regulations.
- Iterate and optimize: Generative AI evolves rapidly, so continually monitor and refine its integration within your workflows. Use performance metrics to assess its impact and identify areas for improvement, ensuring it delivers consistent value to your organization.
- Collaborate across teams: Encourage collaboration between data professionals, domain experts, and decision-makers. Generative AI thrives when diverse expertise comes together to shape its use cases and interpret its outputs effectively.
Tools and technologies to consider
For seamless integration of generative AI into your workflows, consider the following:
- Spotfire® solutions: With an AI-driven recommendations engine and advanced visualizations, Spotfire empowers users to combine AI with powerful data wrangling and predictive analytics tools. Its built-in R and Python support allows customization for domain-specific challenges. With an added generative AI extension, users can ask and answer questions of their data and analysis.
- In-house AI frameworks: Many organizations develop their own AI frameworks tailored to their specific needs. These frameworks can include generative AI components for creating synthetic data or automating repetitive tasks.
- Cloud-based AI services: Leverage AI models and infrastructure available through cloud providers to scale generative AI capabilities without significant hardware investments.
- APIs for generative models: Use APIs for text, image, or data generation to incorporate generative AI outputs directly into analytics workflows. These can be paired with platforms like Spotfire to visualize and analyze the results.
These tools help bridge the gap between generative AI's potential and real-world applications, allowing you to innovate while effectively leveraging existing resources.
Key takeaways
Generative AI is revolutionizing how businesses and data professionals approach creativity and problem-solving. Its ability to generate, simulate, and innovate complements traditional AI's predictive prowess. As data professionals, embracing generative AI is essential to staying competitive in today's fast-evolving landscape.
Explore how Spotfire enhances your AI capabilities and drives better data-driven decision-making.
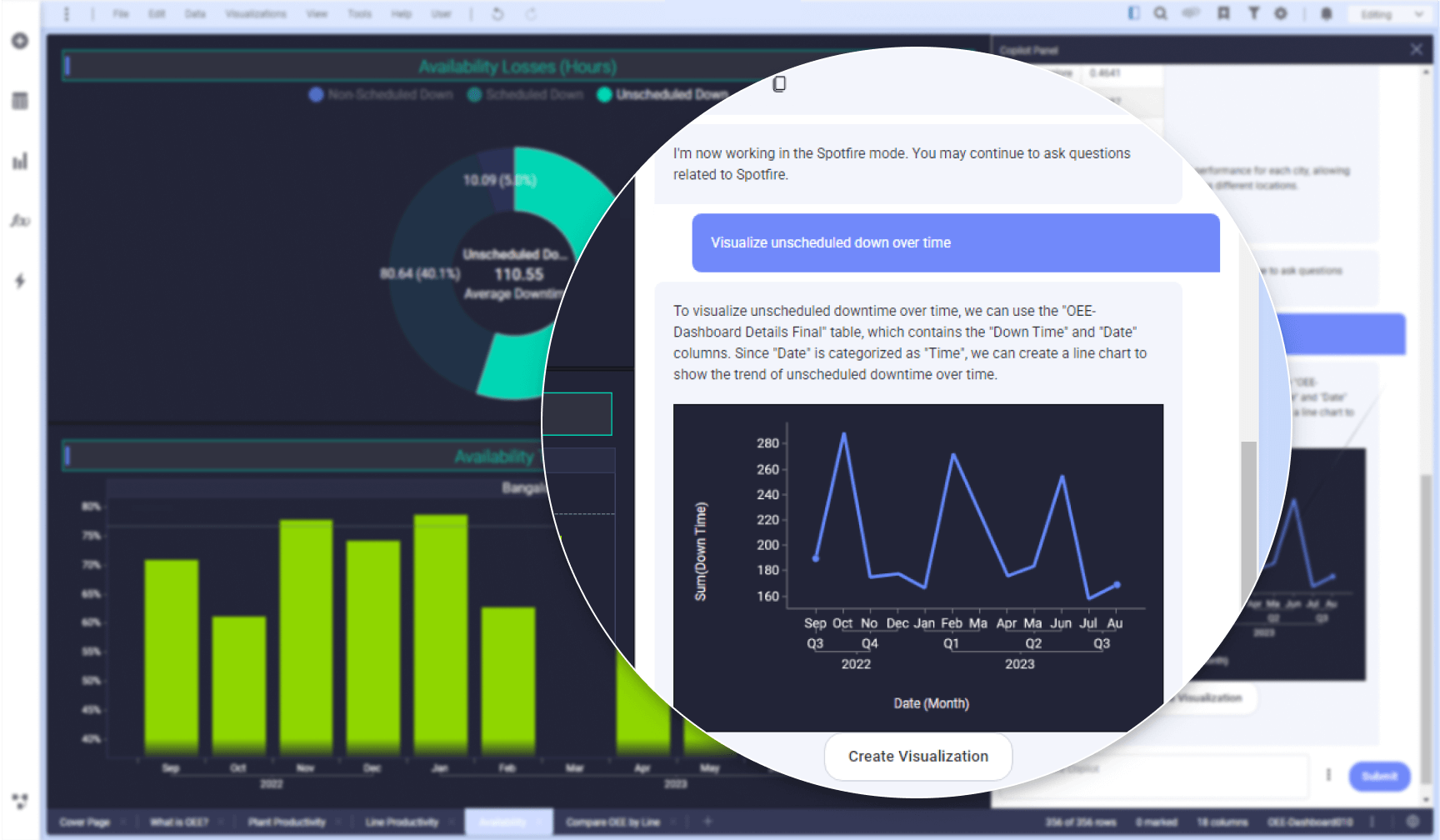
Related resources
Turn predictive findings into optimal outcomes with the latest advancements in AI and ML.
Generative AI is available in Spotfire as an extension. Discover the latest updates in the AI tool, available on the Spotfire Community.
Want to experience generative AI in action within your Spotfire analysis? Download the Spotfire Copilot tool, available on the Spotfire Community.